Neural networks: extensive computer models are tamed
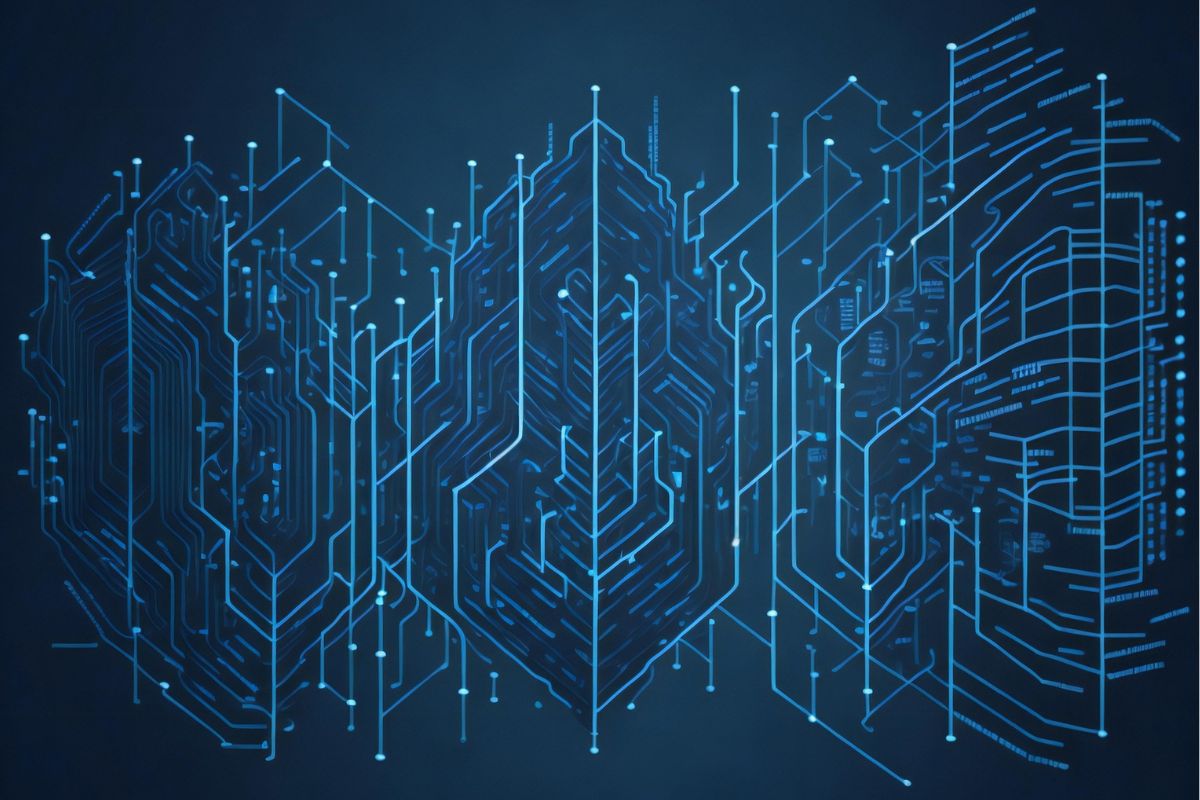
The scientists Prof. Dr. Mehdi B. Tahoori, Soyed Tuhin Ahmed and Christopher Münch from the Chair of Dependable Nano Computing (CDNC) at KIT and their colleagues from the University of Grénoble-Alpes(Guillaume Prenat, Kamal Danouchi, Lorena Anghel) aim to improve the reliability and performance of Bayesian neural networks by using novel spintronic devices and an algorithm-to-hardware approach. They describe their proposed solution in their paper "SpinDrop: Dropout-Based Bayesian Binary Neural Networks with Spintronic Implementation", which was recognized as the best paper by the IEEE Journal on Emerging and Selected Topics in Circuits and Systems.
The implementation of Bayesian networks is a promising way to improve the fault detection and prediction accuracy of neural networks, especially in safety-critical applications such as medicine and the automotive sector. However, conventionalöHowever, traditional implementations of Bayesian networks struggle to work efficiently on small devices (so-called edge AI) and in fast applications. The proposed hardware co-design and co-optimization algorithm can address these problems. This would not only improve the reliability and performance of Bayesian networks, but also positively influence their accuracy in predictions.
The IEEE Circuit and System Society (CAS) Best Paper Award is presented annually to authors of scientific papers that convince the jury with their writing style, the quality of their research and the relevance of their work for practicing professionals.